回歸方程的函數(shù)形式
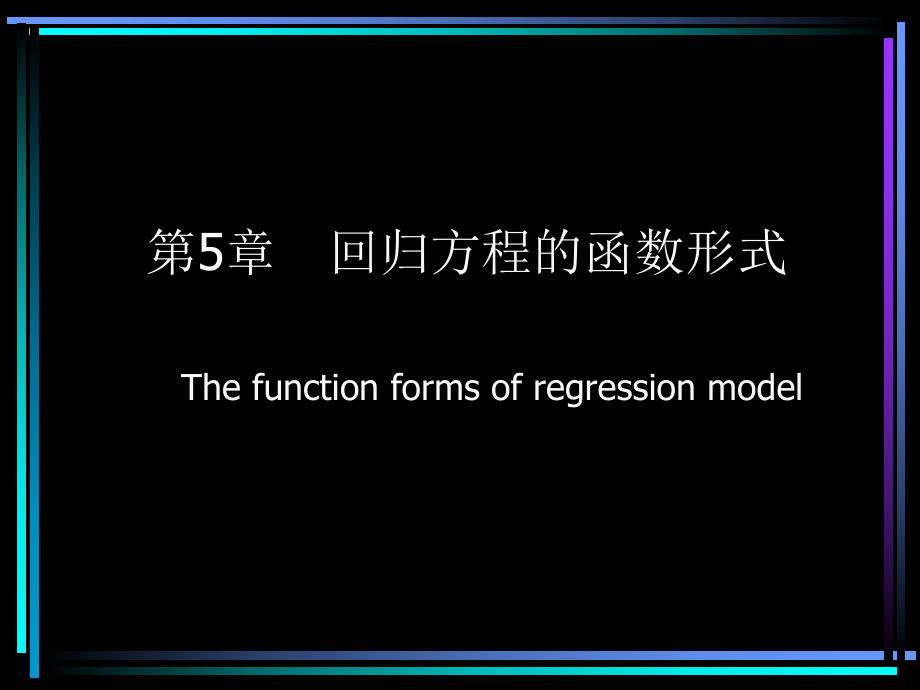


《回歸方程的函數(shù)形式》由會(huì)員分享,可在線閱讀,更多相關(guān)《回歸方程的函數(shù)形式(37頁珍藏版)》請(qǐng)?jiān)谘b配圖網(wǎng)上搜索。
1、第 5章 回 歸 方 程 的 函 數(shù) 形 式The function forms of regression model Contents Log-linear model: measure elasticity (double log model) Semi-log modelLog dependent variable: measure growth (log-lin model)Log independent variables (lin-log model) Hyperbolic model Polynomial model summary Log-linear model:Measu
2、re elasticity 1 21 2 1 2Sometimes, well meet the model like the following:Then How to estimate the model in OLS?You see, in the model, dependent variable is notlinear with the parameters and .uY AX X e Y Log-linear model:Measure elasticity 1 1 2 2 * * *0 1 1 2 2*But, if we transfrom the model by log
3、 two sides simutaneously, we get a new model as following:ln ln ln lnNow, we denote ln , ln , ln , lnThen we can rewrite the model asY A X X uY Y A X X X XY * *0 1 1 2 2which is just general multiple regression modelwhat we have learned in last chapter.X X u Log-linear model:Measure elasticity 0 1 1
4、 2 2 ln ln lnThe model above is often called In the log-linear model, the partialcoefficients are the elasticity of over .For example, in the mY X X uY Xs log-linear model or double log model. 11 1 1 1 1 11 1odel above,ln lnwhich just denote the elasticity of over .That is, change 1%, will change %.
5、d Y XdY Y dYd X dX X dX YY XX Y Log-linear model: example In the end of 1920s, American mathematician Charles Cobb and economist Paul Dauglas put forward the famous production function, that is, Cobb-Dauglas production function. The C-D production function is as following Y = ALaK, the econometric m
6、odel will be Y = ALaK eu Where Y denote the production, GDP, for example, L denote labor input to the production, and K denote total capital. a is the production elasticity of labor, is the production elasticity of capital. Log-linear model: example Y = ALaK 1 1We cant estimate the model directly, b
7、ut wecan transform the model to log-linear model.ln ln ln ln where is the error term and is normdY dL dY L AL K L YY L dL YdY dK dY K AL K K YY K dK YY A L K uu a a a a a al distribution.Then we can use OLS to estimate the new model.Just the production elasticity of laborJust the production elastici
8、ty of capital Example : the original datayear Y L K year Y L K1955 114043 9310 182113 1965 212323 11746 3157151956 120410 8529 193749 1966 226977 11521 3376421957 134705 8738 205192 1967 241194 11540 3635991958 129187 8952 215130 1968 260881 12066 3918471959 139960 9171 225021 1969 277498 12297 4223
9、821960 150511 9569 237026 1970 296530 12955 4550491961 157897 9527 248897 1971 306712 13338 4846771962 165286 9662 260661 1972 329030 13738 5205531963 178491 10334 275466 1973 354057 15924 5615311964 199457 10981 295387 1974 374977 14154 609825 Example (textbook, ex5.2, p105): the transformed dataye
10、ar ln(Y) ln(L) ln(K) year ln(Y) ln(L) ln(K)1955 11.64 9.14 12.11 1965 12.27 9.37 12.66 1956 11.70 9.05 12.17 1966 12.33 9.35 12.73 1957 11.81 9.08 12.23 1967 12.39 9.35 12.80 1958 11.77 9.10 12.28 1968 12.47 9.40 12.88 1959 11.85 9.12 12.32 1969 12.53 9.42 12.95 1960 11.92 9.17 12.38 1970 12.60 9.47
11、 13.03 1961 11.97 9.16 12.42 1971 12.63 9.50 13.09 1962 12.02 9.18 12.47 1972 12.70 9.53 13.16 1963 12.09 9.24 12.53 1973 12.78 9.68 13.24 1964 12.20 9.30 12.60 1974 12.83 9.56 13.32 Example (textbook, ex5.2,): the estimated regression The estimated model: ln() = -1.652 + 0.3397 ln(L) +0.846 ln(K) s
12、e = (0.606) (0.186) (0.093) t = (-2.73) (1.83) (9.06) p = (0.014) (0.085) (0.000) n=20, R2=0.9951 Adj-R2=0.9945 F=1719.46 Remark: The production elasticity of labor is 0.3397, that is, if labor increase 1%,the production will increase 0.3397%. In the same way, the production elasticity of capital is
13、 0.846, thats, if capital increase 1%, the production will increse 0.846%. Example 5.3 Demand for energy: The original datayear demand gdp price year demand gdp price1960 54.1 54.1 111.9 1972 97.2 94.3 95.61961 55.4 56.4 112.4 1973 100 100 1001962 58.5 59.4 111.1 1974 97.3 101.4 120.11963 61.7 62.1
14、110.2 1975 93.5 100.5 1311964 63.6 65.9 109 1976 99.1 105.3 129.61965 66.8 69.5 108.3 1977 100.9 109.9 137.71966 70.3 73.2 105.3 1978 103.9 114.4 133.71967 73.5 75.7 105.4 1979 106.9 118.3 144.5 1968 78.3 79.9 104.3 1980 101.2 119.6 1791969 83.3 83.8 101.7 1981 98.1 121.1 189.41970 88.9 86.2 97.7 19
15、82 95.6 120.6 190.91971 91.8 89.8 100.3 Example 5.3 Demand for energy(textbook, p106) Example 5.3 give the data about the energy demand of seven OECD countries during 19601982. In the table, demand is the total demand for energy, gdp for real GDP, and price for real energy price. Now, we want to est
16、imate the energy demand function. Here, we use the log-linear model as following: ln(demand) = 0 1 ln(gdp) + 2 ln(price) + u 1 is the income elasticity of demand.2 is the price elasticity of demand Example 5.3: the estimated regression The estimated model: ln(demand) =1.54950.9972 ln(gdp)0.3315ln(pr
17、ice) se =(0.0901) (0.0191) (0.0243) t =(17.20) (52.17) (-13.63) p =(0.000) (0.000) (0.000) n=23 R2=0.994 Adj-R2=0.9935 F=1693.67 Remark: The energy demand is positive related with real GDP and negative related with energy price, which accord with the economic theory. The demand elasticity of income
18、is 0.9972, that is, when other factors fixed, if real GDP increase 1%, the demand for energy will increase 0.9972%. The demand elasticity of price is -0.3315, which means when income is fixed, if the price for energy increase 1%, the demand for energy will decrease 0.3315%. Semi-log model There are
19、two kinds of semi- log model: the dependent variable is logged and the independent variables is logged. log dependent variable (log-lin model)ln(Y) = 0 1 X1 + 2 X2 + u log independent variables (lin-log model)Y = 0 1 ln(X1) + 2 lnX2) + u Log dependent variable:measure growth ratew See a simple regre
20、ssion model ln(Y) = 0 1 X + uw What does the coefficient of X mean? We know that 1 is partial deviation of ln(Y) to X, that is, So, the 1 means that independent variable X change 1 unit, the variable Y will change 1001 %. Note here the X is absolute value and Y is the relative value. That is, 1 is t
21、he growth rate of Y when X change 1 unit. 1 lnd Y dY YdX dX Example: wage determinationwThe estimated model: log(wage)=0.2840.092educ+0.0041exper+0.022tenure se = (0.1042)(0.0920) (0.0041) (0.0031) t = (2.73) (12.56) (2.39) (7.13) n=526 R2=0.3160 Adj-R2=0.3121 F=80.39w Remark: The partial coefficien
22、t for educ is 0.092, which means when the education increase 1 year with exper and tenure fixed, then the wage per hour will increase The same meaning to the partial coefficients for exper and tenure. 9.2%. Example: 1960-1982 G DP growthw Let Y0 stand for the value of GDP in 1960 and assume the aver
23、age GDP growth rate is r, then the GDP in 1961 will be Y1=Y0(1+r), the GDP in 1962 will be Y2=Y1(1+r)=Y0(1+r)2, in the same way, the GDP for t year beyond the initial year will be Yt=Y0(1+r)t. (*)w Now, we have the data for GDP (Y) during 19601982. (see example 5.3), we want to estimate the average
24、growth rate of GDP during the period. What should we do? We logged equation (*) two sides simultaneously, and get, ln(Yt)=ln(Y0) + tln(1+r) Example: 1960-1982 G DP growthwThe corresponding econometric model is ln(Yt)=ln(Y0) + tln(1+r) + u let 0 = ln(Y0), and 1= ln(1+r)rwThe the model can rewrite as
25、ln(Yt)= 0 + 1 t + u Now, you see, the partial coefficient 1 stand for the growth of Y when t change 1 unit. That is, 1 is just the growth rate of real GDP. The data for real G DP (Yt)year t gdp year t gdp year t gdp1960 0 54.1 1968 8 79.9 1976 16 105.31961 1 56.4 1969 9 83.8 1977 17 109.91962 2 59.4
26、 1970 10 86.2 1978 18 114.41963 3 62.1 1971 11 89.8 1979 19 118.31964 4 65.9 1972 12 94.3 1980 20 119.61965 5 69.5 1973 13 100 1981 21 121.11966 6 73.2 1974 14 101.4 1982 22 120.6 1967 7 75.7 1975 15 100.5 60 80 100 120 gdp 1960 1965 1970 1975 1980year The scatter between real G DP and t 60 80 100 1
27、20 gdp 0 5 10 15 20 25t Example: 1960-1982 G DP growthw Using the data for real GDP from example 5.3, we estimate the model ln(t)= 4.044 + 0.0382 t se =(0.0158) (0.0012) t = (255.38) (30.97) n=23, R2=0.9786 Adj-R2=0.9776 F=958.96w Remark: The slope of the estimate equation is 0.0382, which means tha
28、t the growth rate for real GDP is 3.82% every year in average. The estimated slope is often called instantaneous growth rate. But we can easily calculate the compound growth rate by ln(1+r)=0.0382, therefore, r=e0.0382-1=1.0389-1=0.0389=3.89%. Linear trend model 60 80 100 120 gdp 0 5 10 15 20 25t w
29、Using the data for real GDP from example 5.3, we estimate the model t= 50.3 + 3.277 t se= (0.776)(0.0566) t = (64.82)(57.90) n=23 R2=0.9938 Adj-R2=0.9935 F=3352.72w Remark: The slope means that t increase 1 unit, the real GDP will inrease 3.277, which is absolute value. That is, real GPD in this yea
30、r will 3.277 greater than that of the year before this year.Linear trend model Sometimes, we meet the model with independent variables logged, such asY = 0 1 ln(X1) + 2 lnX2) + u Then what are the meaning of the partial coefficients?For example, So, 1 means that when independent variable X1 changes
31、1%, the dependent variable will change Note, the change of X1 is relative value and Y is absolute value. 11 1 1 1 1 1, ln dXdY dY dYd X dX X X 1/100. The relation between USA GNP (gnp) and Money supply (m2)gnp = 0 1 ln(m2) + u we estimate the modelgnp = 16329.21 + 2584.785 ln(m2)Se = (696.60) (94.04
32、14)t = (-23.44) (27.49)n=15 R2=0.9831 Adj-R2=0.9818 F=755.46 Remark:The slope means when money supply m2 increase 1%, the USA GNP gnp will increase 2584.785/100= 25.85 Hyperbolic model The hyperbolic model is like the followingY = 0 1 1/X + u Where X is nonlinear with Y, but Y is still linear with t
33、he parameters, so the OLS still work. But instead, we will take 1/X as a new independent variable. In the hyperbolic model, 0 is the asymptotic value or limited value of Y. Hyperbolic model, cont.u = 0 1 1/Xu With different sign for the coefficient, the estimated equation will have different curve.
34、XY 001 00 XY 000Average fixed cost function Engel expenditure curve Philips curveXY 00, 1 0, so when unemployment rate increase, the income growth rate will decrease, which meet the theory. v TC= 0 1 Q + 2 Q2 + 3 Q3 + u TC Qcostv In order to best fit the data, polynomial model may be a good choice.v
35、 For example, we can use model the total cost function as polynomial regression. vJust now, we use the hyperbolic function to model the Philips curve, now we use a polynomial function to remodel the Philips curve. Now, we specify the model as Y=0 1 X + 2 X2 + u v Use the same data in table 5-6, we e
36、stimate the polynomial model above. =23.53-7.24 X + 0.34 X2 se=(3.76) (1.54) (0.15) t = (6.26) (-4.71) (4.22) N=12 R2=0.8370 Adj-R2=0.8228 F=23.11v Using hyperbolic model, estimation of the American Philips Curve is = -0.2594 +20.5879 1/X se=(1.0086) (4.6795) t = (-0.26) (4.40) n=12 R 2=0.6594 Adj-R
37、2=0.6253 F=19.36 v Let see the scatter and fitted curve using polynomial model: 3 4 5 6 7 Y(%)/F itted va lues 3 4 5 6 7X(%)Y(%) Fitted values vBecause there is no intercept, the OLS estimate will be 22 222 , var ,1i ii i iXYX Xen vSeveral important characteristics d.f. of sigma hat squred is n-k (n
38、ot n-k-1), R-squared formula we mentioned before is not eligible in this case. However, we can still use squared the correlation coefficient of Y and its fitted value (Y hat). The sum of squared residuals is not zero. vUsually, unit is not a problem in estimation.vHowever, because of computer calcul
39、ation system, the scale of independent variables and dependent variable should be similar. v The meaning of OLS estimate is how much standard deviation of Y changes when X change one standard deviation.* *0 1* * * *1Standardized variables ,The origion model The nomalized model ii iY Xi i ii i i X XY
40、 YY XS SY X uY X u summary Log-linear model: measure elasticity ln(Y 0 1 ln(X1) +2ln(X2) + u 1 % Semi-log model Log dependent variable: measure growth ln(Y 0 1 X1 +2X2 + u 100 1 % Log independent variables Y 0 1 ln(X1) +2ln(X2) + u 1 /100 Hyperbolic model Y 0 1 1/X1 + u Polynomial model Y 0 1 X +2X2 + u
- 溫馨提示:
1: 本站所有資源如無特殊說明,都需要本地電腦安裝OFFICE2007和PDF閱讀器。圖紙軟件為CAD,CAXA,PROE,UG,SolidWorks等.壓縮文件請(qǐng)下載最新的WinRAR軟件解壓。
2: 本站的文檔不包含任何第三方提供的附件圖紙等,如果需要附件,請(qǐng)聯(lián)系上傳者。文件的所有權(quán)益歸上傳用戶所有。
3.本站RAR壓縮包中若帶圖紙,網(wǎng)頁內(nèi)容里面會(huì)有圖紙預(yù)覽,若沒有圖紙預(yù)覽就沒有圖紙。
4. 未經(jīng)權(quán)益所有人同意不得將文件中的內(nèi)容挪作商業(yè)或盈利用途。
5. 裝配圖網(wǎng)僅提供信息存儲(chǔ)空間,僅對(duì)用戶上傳內(nèi)容的表現(xiàn)方式做保護(hù)處理,對(duì)用戶上傳分享的文檔內(nèi)容本身不做任何修改或編輯,并不能對(duì)任何下載內(nèi)容負(fù)責(zé)。
6. 下載文件中如有侵權(quán)或不適當(dāng)內(nèi)容,請(qǐng)與我們聯(lián)系,我們立即糾正。
7. 本站不保證下載資源的準(zhǔn)確性、安全性和完整性, 同時(shí)也不承擔(dān)用戶因使用這些下載資源對(duì)自己和他人造成任何形式的傷害或損失。
最新文檔
- 6.煤礦安全生產(chǎn)科普知識(shí)競(jìng)賽題含答案
- 2.煤礦爆破工技能鑒定試題含答案
- 3.爆破工培訓(xùn)考試試題含答案
- 2.煤礦安全監(jiān)察人員模擬考試題庫試卷含答案
- 3.金屬非金屬礦山安全管理人員(地下礦山)安全生產(chǎn)模擬考試題庫試卷含答案
- 4.煤礦特種作業(yè)人員井下電鉗工模擬考試題庫試卷含答案
- 1 煤礦安全生產(chǎn)及管理知識(shí)測(cè)試題庫及答案
- 2 各種煤礦安全考試試題含答案
- 1 煤礦安全檢查考試題
- 1 井下放炮員練習(xí)題含答案
- 2煤礦安全監(jiān)測(cè)工種技術(shù)比武題庫含解析
- 1 礦山應(yīng)急救援安全知識(shí)競(jìng)賽試題
- 1 礦井泵工考試練習(xí)題含答案
- 2煤礦爆破工考試復(fù)習(xí)題含答案
- 1 各種煤礦安全考試試題含答案